April 14th, 2025
Category: Uncategorized
No Comments
Posted by: Team TA
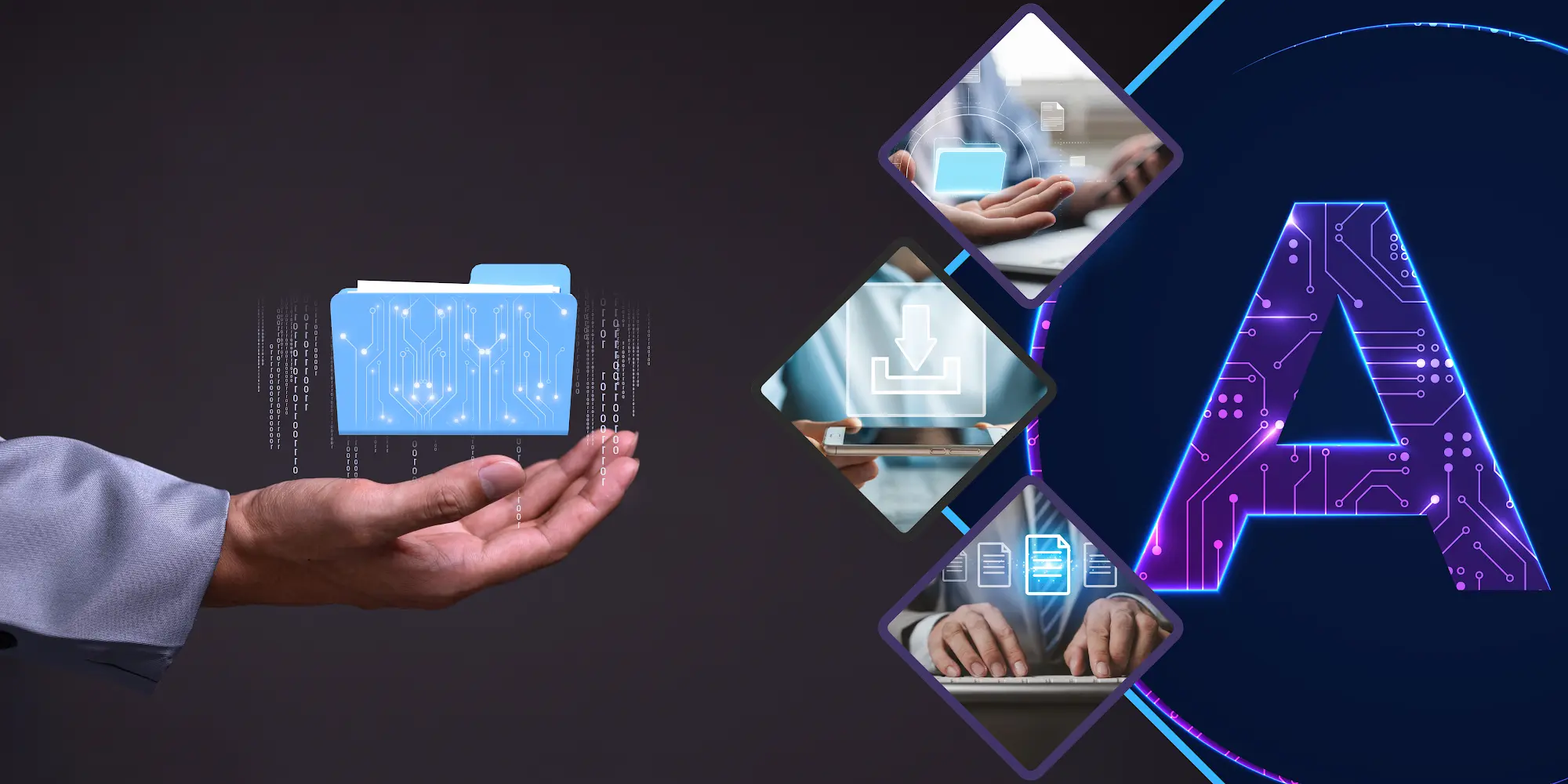
With AI adoption growing rapidly, industries are generating vast amounts of data that require immediate analysis. Traditional cloud computing faces limitations in handling this demand efficiently, leading to the rise of AI and Edge Computing. By processing data closer to its source, edge technology lowers latency and boosts efficiency. The global edge computing market is expected to grow to $350 billion by 2027, according to Statista, highlighting its growing importance in industries like telecommunications and manufacturing.
Companies like Amazon, Microsoft, and Google are advancing cloud-edge technologies, making AI-driven applications more accessible and efficient. This article aims to provide insights into the impact of AI Edge Computing, its advantages, real-world applications, and how businesses can adopt this technology. By understanding the synergy between AI and Edge, industries can unlock new opportunities for innovation and growth.
What is Edge Computing and Why Industries are Moving towards it?
Edge computing is a technology that processes data closer to where it is generated, rather than relying on distant cloud servers. With the increasing number of connected devices, the need for faster computing with low latency is growing. According to the Linux Foundation, edge computing is shaping the “Third Act of the Internet,” where a significant portion of data storage and processing will occur at the network’s edge, improving efficiency and speed.
Edge computing improves performance, decreases latency, and lowers operating costs by enabling local data processing. For instance, smart home devices can process commands directly on the device, guaranteeing faster responses, rather than sending requests to distant servers.
Edge computing is being quickly adopted by industries to increase automation, productivity, and decision-making. The growing need for real-time data processing, particularly in dynamic environments, is too much for traditional computing models to handle. Without requiring continuous cloud connectivity, AI at the Edge enables machines to identify patterns, make decisions, and adjust to changing conditions. For sectors like manufacturing, healthcare, and smart cities, this change is crucial because it allows for quicker reaction times, lower operating costs, and increased security.
How do AI and Edge Computing work together?
By processing data closer to its source, AI and Edge Computing improve real-time decision-making and lower latency. AI Edge Computing allows devices to analyze data locally, in contrast to traditional cloud computing, which sends data to centralized servers. In sectors like IoT, healthcare, and smart manufacturing, this improves efficiency and speeds up reaction times. IDC predicts that by 2026, 75% of major enterprises will use edge computing driven by AI to enhance product quality and optimize supply chains.
Businesses can reduce network congestion and improve security by incorporating artificial intelligence at the edge. AI uses machine learning and sophisticated analytics to process information locally rather than sending large amounts of data to the cloud. In addition, IDC projects that machine learning will be used in 50% of edge computing deployments by 2026, up from 5% in 2022, underscoring the expanding role of AI in edge computing.
Advantages of AI Edge Computing
- Smarter and More Adaptive AI
Unlike traditional applications, AI Edge Computing does not rely on preset inputs but instead uses neural networks to analyze and respond to new and varied data types, including text, voice, and video. AI is flexible enough to learn and evolve without demanding regular programming updates.
- Easy Scalability
Edge AI expands to meet business demands. Businesses can increase their AI capabilities by adding more edge devices rather than updating centralized servers. This ensures the smooth integration of new technologies and makes scaling economical and effective.
- Stronger Privacy Protection
Local processing ensures the security of private data, including voice recordings, security footage, and medical images. The risk of data breaches and unauthorized access is decreased because only analyzed insights are sent to the cloud.
- Lower Costs
By processing data locally, edge AI reduces dependency on the cloud, resulting in lower bandwidth and storage costs. Additionally, automated AI systems cut operating costs by eliminating the need for human oversight.
- Improved Data Security
Cyberattacks are less likely to occur during data transmission when information is processed closer to the source. Maintaining sensitive data on the device improves security and complies with privacy laws.
- Real-Time Performance
Edge AI is ideal for time-sensitive applications such as manufacturing defect detection or security threat monitoring because it processes data instantly. Because Edge AI avoids cloud delays, it ensures faster reaction times and greater operational efficiency.
- Energy Efficiency
By processing data locally and removing the need to send massive amounts of data to cloud servers, AI Edge Computing lowers power consumption. The energy-efficient design of edge devices results in reduced electricity costs and a smaller carbon footprint.
- Uninterrupted Functionality
Edge AI allows devices to function independently, even in remote or unconnected locations. In contrast to cloud-based systems that depend on constant internet access, edge devices guarantee high availability for vital applications.
Comparing the 5 Leading AI Edge Computing Devices
Devices | AI Performance | Processor | Key Features | Use Cases |
---|---|---|---|---|
NVIDIA Jetson Xavier NX | Up to 21 TOPS | 3-core NVIDIA Volta GPU, 6-core ARM CPU | Compact, integrates with NVIDIA JetPack SDK, supports multiple sensors | Natural language processing, robotics, computer vision |
Google Coral Dev Board | 4 TOPS | Quad-core CPU | Edge TPU for minimal power consumption, 1GB RAM, supports TensorFlow Lite, multiple I/O ports | Object detection, image recognition |
Raspberry Pi 4 | N/A | Quad-core ARM Cortex-A72 CPU | Affordable, supports up to 8GB RAM, Ethernet, USB 3.0, dual 4K HDMI output | Automation, industrial control, IoT |
AWS DeepLens | AI-powered video analytics | Intel Atom processor | Integrated HD camera, AWS IoT Greengrass, AWS integration for local AI inference | AI video analytics, reducing cloud reliance |
Intel NUC | N/A | Intel Core i3 to i7 processors | Compact, up to 64GB RAM, Thunderbolt 3 connectivity, supports IoT gateways, automation, digital signage | IoT gateways, automation, digital signage |
Cost Factors of Building an AI Edge Strategy
- Infrastructure costs
- Sensors
The type and number of sensors required determine the cost of implementing AI at the edge. IoT sensors are already widely used by businesses, which lowers additional costs. But adding specialized sensors—like cameras, microphones, barcode scanners, and RFID tags—can have a big financial impact. Cameras are especially beneficial because they are affordable and can support several AI applications at once.
- Computing Systems
Low-cost embedded devices and high-performance servers are examples of edge computing systems. The amount of computing power needed depends on the volume of data, the complexity of the AI model, and the demands of real-time processing. Multi-GPU configurations provide superior performance for running multiple AI applications, optimizing both cost and space, even though single GPU systems might be initially more affordable.
- Network
Most enterprise-edge applications operate for free on local wired or wireless networks. Nevertheless, cellular networks can result in high data transmission costs for distant devices, particularly when handling video. To reduce expenses and enhance AI model performance, businesses must also consider the requirements for data transfer between edge locations and cloud servers.
- Application costs
- Developing AI Applications
Creating AI applications internally requires a skilled data science team, which can be expensive and time-consuming. Hiring AI developers typically entails a high salary range of $100K to $150K per expert. Many companies employ a hybrid approach, purchasing prebuilt solutions for non-essential tasks while creating essential AI applications internally.
- Purchasing AI Applications
Purchasing AI applications is a sensible option for businesses without AI experts. Depending on licensing, prebuilt applications can cost anywhere from thousands to tens of thousands of dollars and offer customization options. To ensure long-term effectiveness, businesses can also spend money on service contracts for continuing maintenance, updates, and support.
- Management costs
- Edge AI Management Software
The management of edge AI environments can be challenging due to their remote deployment and the lack of on-site IT staff. Organizations can purchase dedicated management solutions, like NVIDIA Fleet Command, Azure IoT, or AWS IoT, which vary in price based on usage and scalability. As an alternative, licensing more conventional data center tools like VMware Tanzu to the Edge or RedHat OpenShift may be more expensive.
- Outsourced Management Services
System integrators can be used by businesses with little internal experience to outsource management. Depending on their extent, these services can cost anywhere from hundreds of thousands to millions of dollars and include AI model development, infrastructure management, and updates.
The Impact of AI and Edge Computing in Manufacturing
By combining operational technology (OT) and information technology (IT) for increased productivity and real-time decision-making, AI and Edge Computing are revolutionizing manufacturing. Manufacturers require ways to improve automation, streamline supply chains, and cut downtime while maintaining sustainability. Predictive analytics driven by AI improves reliability and lowers waste by identifying problems before they affect production.
Real-time monitoring, automation, and machine-to-machine communication are made possible by edge computing, which processes data closer to the factory floor. This helps manufacturers in tracking machine performance, scanning materials, and improving logistics without cloud delays.
Use Cases of Edge Computing in Manufacturing
1. Predictive Maintenance
Using AI and sensor data, edge computing makes it possible to monitor machinery in real-time. Early equipment failure detection allows factories to avoid expensive downtime. For instance, chemical plants can detect pipe corrosion with sensor-equipped cameras, enabling prompt repairs.
2. Inspection and Quality Control
Manufacturing factories must identify flaws quickly to maintain high product standards. Edge computing makes it possible for machines to identify defects in a matter of microseconds and alert staff by processing data locally. This reduces waste and boosts production efficiency, ensuring that only high-quality products reach the market.
3. Optimization of Equipment
By analyzing sensor data, manufacturers can evaluate overall equipment effectiveness (OEE) in real-time. For instance, automotive welding flaws can be immediately identified before products leave the factory by utilizing AI in edge computing.
4. Factory Floor Optimization
Edge AI analyzes worker movements and machine placements to identify inefficiencies. In car manufacturing, for example, it can help optimize worker paths, reducing unnecessary movement and improving workflow.
5. Supply Chain Analytics
With automated inventory tracking, manufacturers can predict shortages and adjust production instantly. An electronics company, for example, can use edge AI to signal other factories to produce more raw materials, preventing supply chain disruptions.
6. Safer Work Environment
Edge AI-powered cameras and sensors monitor real-time workplace safety. Businesses can ensure a safer working environment by taking prompt action to prevent accidents by identifying hazardous conditions or improper use of heavy machinery.
The Impact of AI and Edge Computing in Media and Entertainment
The combination of Artificial Intelligence (AI) and Edge Computing is profoundly transforming the media and entertainment industry, driving innovation in content creation, distribution, and consumption. AI-powered tools enable real-time content creation and editing, allowing media professionals to streamline workflows by automating tasks such as video editing, visual enhancements, and subtitle generation. With edge computing, these processes can be executed locally, reducing latency and enabling faster content turnaround. AI and edge computing also enhance user experiences by personalizing content recommendations based on real-time analysis of user preferences, ensuring that consumers enjoy tailored and immersive experiences. This is particularly evident in streaming platforms, where AI helps deliver personalized content and edge computing ensures low-latency performance for smooth delivery, even during live events.
Moreover, AI and edge computing play a crucial role in content localization by automating translation, dubbing, and subtitling, making it easier for media companies to reach global audiences. In post-production, these technologies enable automation in tasks such as color correction, audio mixing, and visual effects, freeing creative professionals to focus on the artistic aspects of content. Additionally, AI-driven content discovery systems improve search functions, making it easier for users to find content suited to their tastes, while edge computing ensures fast processing and reduced network congestion.
Security and piracy prevention also benefit from AI and edge computing, with AI algorithms detecting and preventing unauthorized distribution of content in real time. The decentralization of processing through edge devices further enhances content security by minimizing exposure during transit. For live event streaming, AI and edge computing deliver real-time video optimization, audience interaction, and personalized viewing experiences. This is especially valuable for sports, concerts, and e-sports, where real-time analytics improve engagement and viewer satisfaction.
Finally, the cost efficiency and scalability enabled by edge computing are significant for media companies. By reducing reliance on centralized cloud infrastructure, companies can lower operational costs and scale more efficiently, managing increased demand without overwhelming centralized servers. In conclusion, the integration of AI and edge computing is revolutionizing the media and entertainment sector, offering faster, more efficient workflows, personalized user experiences, and enhanced security, while providing new opportunities for growth and innovation.
Use Cases of Edge Computing in Media and Entertainment
- Real-Time Content Delivery & Streaming Optimization
Edge computing reduces latency by bringing content closer to the user. This enables:
- Ultra-low-latency live video streaming
- Seamless 4K/8K video playback
- Real-time adaptive bitrate streaming
- Augmented Reality (AR) & Virtual Reality (VR) Experiences
Edge infrastructure processes heavy data near the user, enabling:
- Immersive, interactive AR/VR content
- Real-time rendering for gaming and virtual concerts
- Location-specific AR advertising and experiences
- Live Event Production and Broadcasting
Edge computing enables localized data processing at stadiums, studios, or on-site events to:
- Streamline multi-camera feeds and instant replays
- Reduce reliance on centralized data centers
- Enable real-time audience interactions and overlays
- Content Personalization and Targeted Advertising
With edge-enabled data processing:
- User behavior is analyzed locally for faster, personalized recommendations
- Real-time, location-based ads can be served efficiently
- Enhances engagement and ROI for advertisers
- Faster Content Creation and Collaboration
For content creators and studios:
- Edge nodes support collaborative editing, rendering, and post-production workflows
- Reduces upload/download times for large media files
- Supports real-time co-editing and remote production setups
Steps for Preparing AI and Edge Computing Integration in Businesses
1. Identify a Use Case
Businesses must have a well-defined use case that supports their objectives—whether they are increasing security, cutting expenses, or increasing efficiency—before deploying AI and Edge Computing. For instance, AI-driven edge solutions in retail can lessen product loss, a problem that costs $100 billion worldwide. Businesses can save billions of dollars with a mere 10% reduction.
Businesses should take important factors into account when choosing the best use case. There should be sufficient business impact to justify the investment. A seamless implementation is ensured by involving stakeholders—IT teams, developers, and system integrators—early in the process. A realistic timeline will ensure AI solutions provide long-term value, while clearly defined success criteria will keep projects on track.
2. Evaluate Your Data and Application Requirements
Data is essential for AI and Edge Computing’s model training and real-time decision-making. Companies must determine what data is required and how it will be used given the billions of sensors at edge locations. To ensure accurate AI predictions, high-quality, labeled data is essential.
Businesses can generate synthetic data when real data is limited, crowdsource data from users, or use internal experts to label data to collect data efficiently. Businesses can proceed with successfully training and implementing AI models as soon as the appropriate data becomes available.
3. Understand Edge Infrastructure Requirements
Setting up AI and Edge Computing requires a robust infrastructure that supports security, bandwidth, and performance. Unlike centralized data centers, edge environments must handle real-time processing while managing space and power limitations.
First, evaluate the existing computing systems, networks, and sensors. Sensors like cameras, radar, and temperature detectors provide data, and computing systems need to balance power and performance demands. For stability, wired connections are ideal, and networks that are reliable and fast are ideal.
4. Roll Out Your Edge AI Solution
An important step before implementing an edge AI application is testing. Before expanding, a proof-of-concept (POC) helps assess performance at a few locations. To make sure the AI system performs well in real-world scenarios, this stage may take several months.
Scalability should be considered while maintaining the original scope’s focus. Flexibility is crucial for AI applications to change with the times while retaining precision and effectiveness.
Top 5 Edge AI Development Companies
1. NVIDIA
NVIDIA is a global leader in AI and edge computing, offering powerful solutions through its Jetson platform. This platform enables businesses to build AI-powered applications for robotics, smart cities, and industrial automation. NVIDIA’s edge AI solutions help process data in real time, reducing latency and enhancing operational efficiency.
2. Intel
Intel provides a wide range of Edge AI hardware and software, including its Intel® processors and AI development tools. These solutions help businesses deploy AI at the edge for industries such as retail, automotive, and IoT, enabling faster decision-making and improved data processing.
3. Qualcomm
Qualcomm specializes in AI-driven processors, such as Snapdragon, which power smartphones, cameras, and automotive applications. Their energy-efficient AI chips optimize real-time analytics on edge devices, improving automation and efficiency.
4. IBM
IBM’s Edge AI platform offers real-time analytics and machine learning solutions for industries like healthcare, finance, and manufacturing. Their AI-driven approach enhances automation and reduces processing delays.
5. Siemens
Siemens provides advanced-edge AI solutions designed for various industries, including manufacturing, transportation, and energy. Their technology combines artificial intelligence with edge devices, allowing for real-time decision-making, prediction of maintenance needs, and increased automation.
Wrapping Up
Edge AI is anticipated to grow rapidly due to advancements in 5G, decentralized computing, and AI automation. Real-time data processing will be enhanced by 5G integration, benefiting sectors like driverless cars and smart cities. Additionally, by facilitating direct data sharing between devices, edge-to-edge collaboration will reduce dependency on the cloud.
This shift will result in faster, smarter, and more autonomous AI solutions across industries. As AI, cloud, and quantum computing continue to advance, companies that use these technologies will have a competitive edge. Industry intelligence, responsiveness, and productivity will all increase due to this change, and automation will also advance, creating new opportunities.